Introduction: From Inboxes to Instant Answers
Imagine this: an employee needs to know the refund process for enterprise clients in Germany. Instead of pinging three departments, scrolling through outdated wikis, or waiting hours for a reply—they simply type the question into a chatbot and get an accurate answer in seconds.
Welcome to the world of internal AI knowledge bases, powered by Large Language Models (LLMs).
In this article, we’ll explore how to design and deploy enterprise-grade internal knowledge assistants, covering everything from RAG pipelines and vector databases to real use cases and security best practices. If you’re looking to scale internal support and reclaim productivity hours, this guide is your starting point.
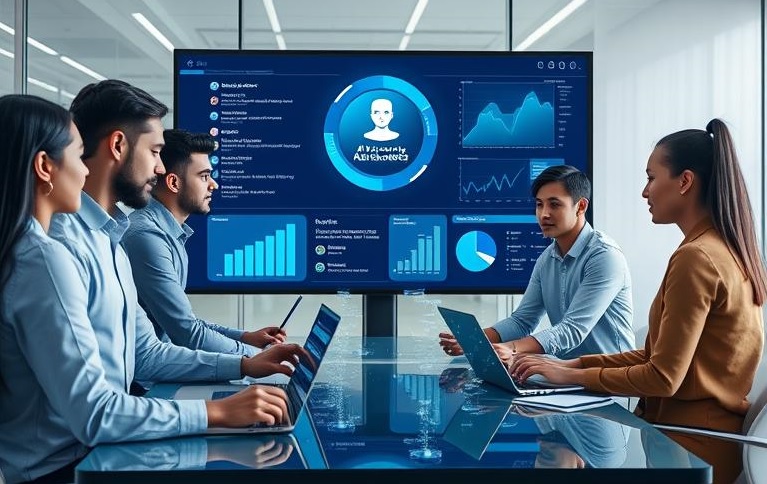
What Is an Internal Knowledge Assistant?
An internal knowledge assistant is an AI-powered tool—often built as a chatbot or API—that answers employee questions by accessing your organization’s private documents, policies, and procedures.
Unlike public models that rely on web knowledge, these assistants use Retrieval-Augmented Generation (RAG) to search internal documents and generate personalized, real-time answers.
What it does:
What it replaces:
Retrieval Techniques: Vector Stores & Embeddings
LLMs don’t “remember” your private data by default—they need retrieval systems to fetch relevant context. That’s where vector stores and embeddings come in.
1. Embeddings:
Embeddings are numeric representations of text. For example, the sentence “How do I request vacation leave?” is converted into a dense vector.
2. Chunking:
Long documents are split into digestible sections (e.g., 200-300 words), so embeddings can be generated efficiently.
3. Vector Stores:
These are databases optimized to store and search vectorized content.
Popular options:
4. Retrieval Flow:
User query → Convert to embedding → Match with closest document chunks → Send results to the LLM → LLM generates answer.
This is the core of a RAG (Retrieval-Augmented Generation) pipeline.
Architecture Overview: LangChain + OpenAI Example
Let’s look at a simplified yet production-ready architecture.
Stack:
Flow:
1. User enters question into chatbot
2. LangChain:
3. LLM generates concise, tone-aligned answer
4. Response is streamed to user
LangChain handles prompt templating, token limits, and routing logic between tools.
Bonus: You can add metadata-based filtering (e.g., by department, date, or source type) to improve relevance.
Enterprise Use Cases That Actually Work
Here are real-world applications where internal AI assistants are making a measurable impact:
1. Internal FAQs & Policy Lookup
Example: “What’s our reimbursement policy for travel over ₹5000?”
Replace static wikis and outdated PDFs with instant answers. Update the source files, and the assistant stays current.
2. IT Helpdesk Bots
Example: “How do I reset my VPN access on a company-issued MacBook?”
Automate 60-70% of repetitive IT queries. Integrate with ticketing tools (like Jira or Freshservice) to escalate complex issues automatically.
3. HR Virtual Assistants
Example: “How many sick leaves are carried over to next year?”
Employees love self-service. HR teams get fewer distractions. Win-win.
4. Compliance & Audit Assistant
Example: “Where is the clause about vendor payment terms in our Q1 supplier agreement?”
Let legal and compliance teams search across contracts, policies, and audit logs securely—without inbox archaeology.
Security and Data Access Tips
Privacy and security are non-negotiable in enterprise deployments.
1. Authentication Layers
Use SSO or OAuth for employee authentication. Ensure each session is tied to an access-controlled identity.
2. Role-Based Access
Define which teams can access which datasets. A junior intern shouldn’t get access to salary band documents.
3. Data Masking
Scrub PII (names, salaries, email addresses) during chunking or before embedding.
4. On-Premise or VPC Deployment
For highly sensitive environments, use open-source models (Mistral, LLaMA 2) with self-hosted infrastructure.
5. Audit Logging
Log every query and response for security review and performance tracking.