Introduction: Great Prompts = Great AI
Crafting the right prompt is like writing a great email subject line—if it’s too vague, too long, or missing the point, you’ll never get the result you want.
As large language models (LLMs) become more integrated into enterprise workflows, the importance of prompt design has never been greater. But here’s the catch: even advanced users often fall into common traps that lead to hallucinations, irrelevant answers, or inconsistent formatting.
In this guide, we’ll unpack the most common prompt engineering errors, walk through real examples and fixes, and leave you with a battle-tested framework for getting the best out of your AI tools.
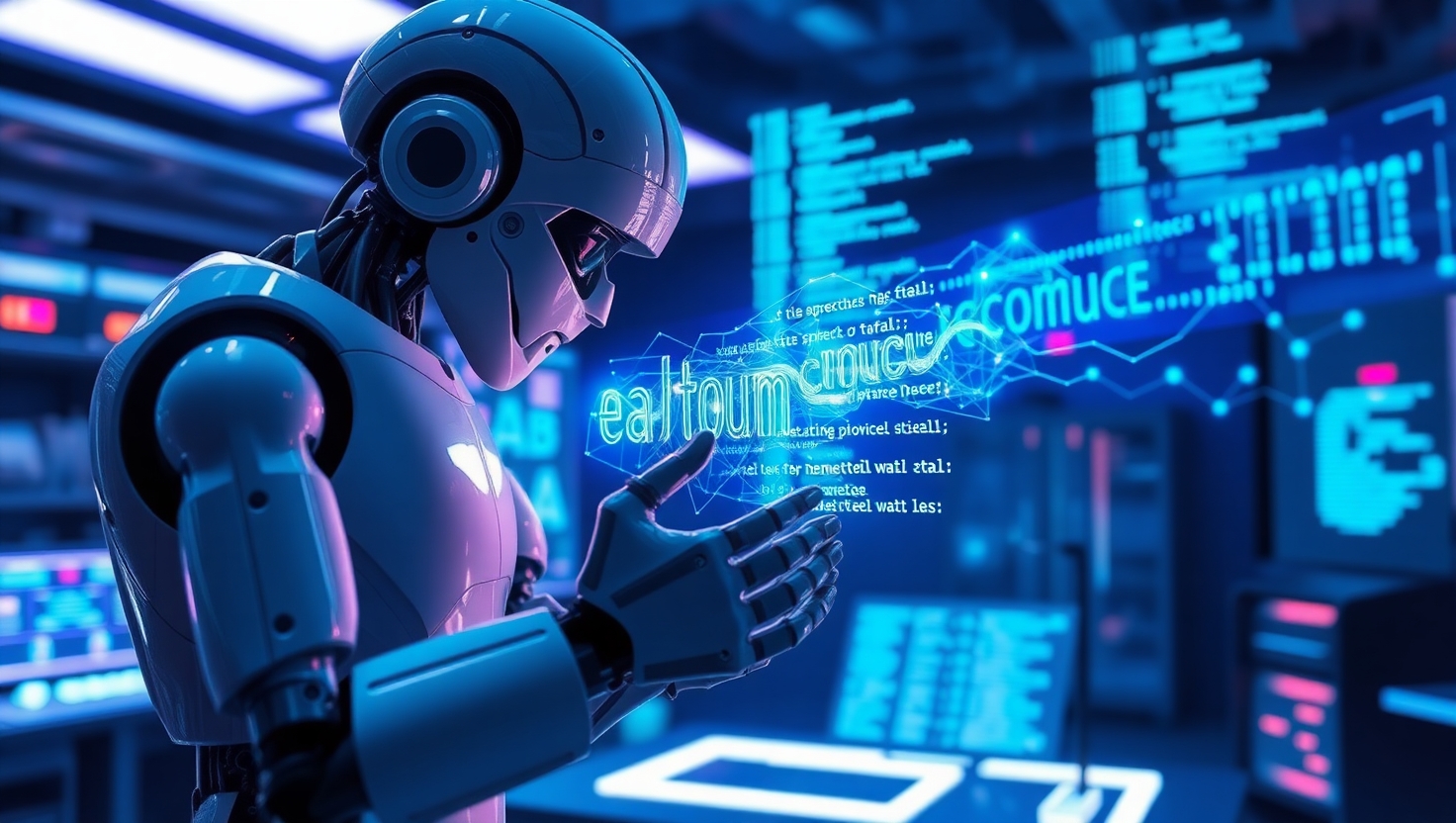
Why Prompt Quality Matters
Think of prompt engineering as talking to an overqualified assistant—one that can do almost anything, but only if you give them crystal-clear directions.
A well-engineered prompt ensures:
Poor prompts, on the other hand, can lead to:
And yet, most of these issues can be traced back to a handful of avoidable mistakes.
Top 5 Prompt Engineering Errors
1. Too Vague or Too Long
The Mistake:
Vague instructions confuse LLMs. Conversely, excessively long, cluttered prompts cause cognitive overload.
Bad Prompt:
“Can you help me with something related to marketing emails?”
Problem:
Fix:
“Write a 100-word promotional email for a fitness app targeting Gen Z users, focusing on a limited-time 30% discount.”
Why it works:
2. No Defined Format in the Output
The Mistake:
You didn’t specify how you want the result structured, so the AI guesses—and usually not in the way you intended.
Bad Prompt:
“List some pros and cons of remote work.”
Fix:
“List 3 pros and 3 cons of remote work in bullet points. Bold the headers.”
Why it works:
3. Ignoring Model Limitations
The Mistake:
Assuming the AI can remember an entire 50-page document or perform multi-step logic without guided reasoning.
Symptoms:
Fixes:
Example:
“Let’s solve this step-by-step. First, calculate the total revenue. Then calculate the profit margin.”
4. Prompt Bloat (a.k.a. Word Salad)
The Mistake:
You try to be overly polite, verbose, or give 5 instructions at once.
Bad Prompt:
“Hi there! I was wondering if you could maybe please help me by writing, if it’s not too much trouble, a blog intro for my post about time management tips…”
Fix:
“Write a 100-word blog introduction on time management tips for remote workers.”
Why it works:
5. Ignoring Output Testing
The Mistake:
You deploy a prompt once and assume it will always perform reliably.
Fix:
Real-life example:
A customer support team used one prompt for refund requests. After A/B testing five variants, one version increased helpfulness ratings by 37%.
Real Examples and Fixes
Let’s break down a few scenarios:
Use Case | Common Error | Fixed Prompt |
Resume Scanning Bot | “Tell me about this candidate.” | “Summarize the candidate’s years of experience, top 3 skills, and relevant industries in 3 bullet points.” |
Product Descriptions | “Describe this product” | “Write a 3-sentence product description for a budget smartphone targeting college students. Include price and battery life.” |
Legal Contract Review | No clause context | “Summarize Clause 4.3 of this employment agreement, focusing on non-compete terms.” |
Best Practices for Clean Prompt Engineering
Here’s a quick checklist to avoid prompt engineering errors:
Be Specific – Define what, how, and for whom.
Define Output Structure – Bullet points, JSON, markdown, etc.
Avoid Redundancy – Clear > Courteous
Break Tasks Down – One step per prompt
Iterate – Review, refine, re-test
Testing Frameworks for Prompt Engineering
Prompt engineering isn’t “set it and forget it.” You need a testbench.
Here’s how to build one:
1. Prompt Versioning
Track changes and outcomes across prompt iterations. Tools like PromptLayer or LangChain help manage this.
2. Gold-Standard Comparisons
Create reference responses. Use them to score LLM outputs on:
3. Prompt Stress Tests
Test how your prompt holds up with:
Final Word: Prompting Is Strategy
AI isn’t just about getting answers—it’s about asking better questions. Whether you’re building a chatbot, automating tasks, or generating reports, mastering prompt engineering means fewer headaches and better results.
Avoiding these common prompt engineering errors can save your team time, reduce costs, and deliver outputs that actually make sense.
The next time your AI output feels “off,” don’t blame the model—check your prompt.