Introduction: Beyond the Hype—Why Evaluation Is Non-Negotiable
So you’ve integrated a large language model (LLM) into your enterprise stack. Maybe it’s powering an internal chatbot, writing marketing content, or summarizing legal contracts. But here’s the million-dollar question:
How do you know it’s actually working?
In a world where LLMs like GPT-4, Claude, or LLaMA are embedded in decision-making, customer interaction, and content generation, LLM performance evaluation isn’t optional—it’s critical.
This guide unpacks how to evaluate your model’s output using the right metrics, tools, and techniques. From factual accuracy to toxicity detection, we’ll cover how to benchmark real-world performance and refine your AI with confidence.
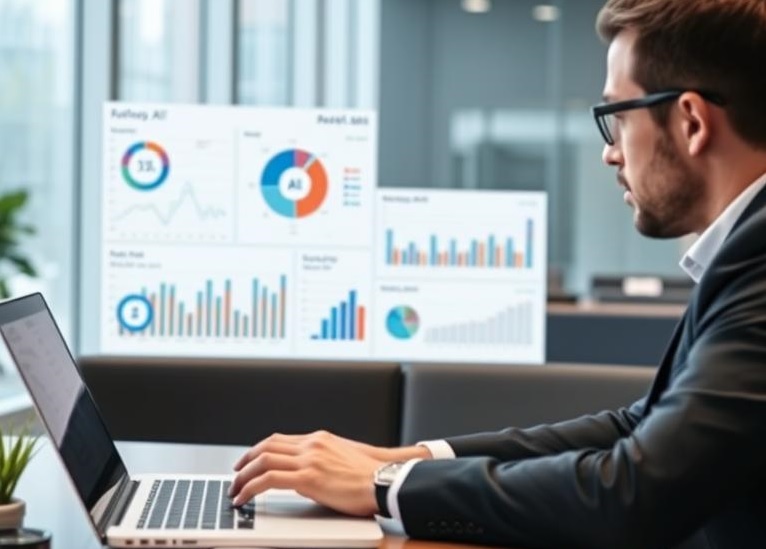
Why LLM Evaluation Matters in Business
Large language models don’t operate in a vacuum. Their outputs influence:
Yet unlike traditional software, LLMs don’t have deterministic outputs. You could input the same question twice and get different answers. That’s why consistent evaluation and tuning are key to reliability.
Bad outputs = bad outcomes. Think:
Your enterprise reputation, customer trust, and operational efficiency depend on getting it right.
Key Metrics to Measure LLM Performance
Let’s break down the core criteria you should track when evaluating LLMs in production.
1. Factual Accuracy
What it means:
Does the model return true, verifiable, and up-to-date information?
Why it matters:
LLMs can “hallucinate”—generating plausible-sounding but false answers. This is dangerous in domains like law, finance, and healthcare.
How to test:
2. Toxicity & Bias
What it means:
Is the output offensive, biased, or harmful in any cultural or demographic context?
Why it matters:
Even subtle bias in hiring bots or customer support assistants can lead to reputational or legal risks.
Tools for toxicity scoring:
3. Response Time / Latency
What it means:
How long does it take for the model to return an answer?
Why it matters:
Speed = user experience. For customer-facing apps, anything above 2–3 seconds feels sluggish.
How to optimize:
4. Relevance & Contextuality
What it means:
Does the output stay relevant to the prompt and business use case?
Why it matters:
Even grammatically perfect answers are useless if they miss the business context.
Example failure:
A model explaining “stock options” from a general finance POV when the user asked about employee stock options.
Human Evaluation vs Auto Scoring
Both approaches have pros and cons—and you’ll often need both.
Human Evaluation
Pros:
Cons:
Auto Scoring
Pros:
Cons:
Best Practice: Use auto-scoring for day-to-day QA and human reviewers for benchmark-setting and high-impact cases.
Tools That Make Evaluation Easier
A few platforms and libraries are leading the way in LLM performance testing:
1. OpenAI Evals
2. Humanloop
3. Trulens
These tools are especially useful in RAG (retrieval-augmented generation) and chatbot scenarios where accuracy, tone, and user experience must all be evaluated continuously.
Post-Evaluation: Optimization Strategies
Once you’ve identified where the LLM falls short, here’s how to fix it.
1. Prompt Tuning
2. Temperature Adjustments
3. Embedding Filtering
4. Hybrid Scoring Systems
Real-World Use Cases
FinTech Chatbot
Scenario: Auto-summarizes loan options for different user profiles
Evaluation Focus: Regulatory compliance, tone neutrality
Tool Used: OpenAI Eval + manual legal review
HR Assistant
Scenario: Answers internal policy questions (leave, benefits)
Evaluation Focus: Factuality, cultural sensitivity
Optimization: Updated HR handbook embeddings + prompt version control
Healthcare LLM
Scenario: Patient symptom explanation
Evaluation Focus: Hallucination risk, liability exposure
Strategy: Every response reviewed by licensed nurse before delivery